The “Goldilocks” Principle: How Do We Get Health AI Regulation “Just Right” to Encourage Innovation and Protect Americans?
2:30 pm
At this pivotal moment, we have a unique opportunity to get AI integration in health care “just right.” Panelists discussed how policymakers can effectively balance advancing new AI technologies with ensuring safety, privacy, and efficacy in health care. Drawing from historical examples, experts shared valuable lessons from past policy implementations, including the acceleration of telehealth adoption, the evolution of HIPAA on data and patient privacy, and the modernization of electronic health records infrastructure.
Speakers
- Moderator
- Damon Davis, MBA, Host, “Discovery Diaries with Damon Davis” podcast
- Panelists
- Deven McGraw, J.D., MPH, LLM, Chief Regulatory and Privacy Officer, Ciitizen Health, Inc.
- Geeta Nayyar, M.D., MBA, Chief Medical Officer, Technologist & WSJ Best selling author, Dead Wrong
- Jeff Smith, MPP, Deputy Director, Certification & Testing Division, Office of the National Coordinator for Health Information Technology, U.S. Department of Health and Human Services
Summit Details
This panel is part of a larger summit event.
July 25, 2024
Speakers
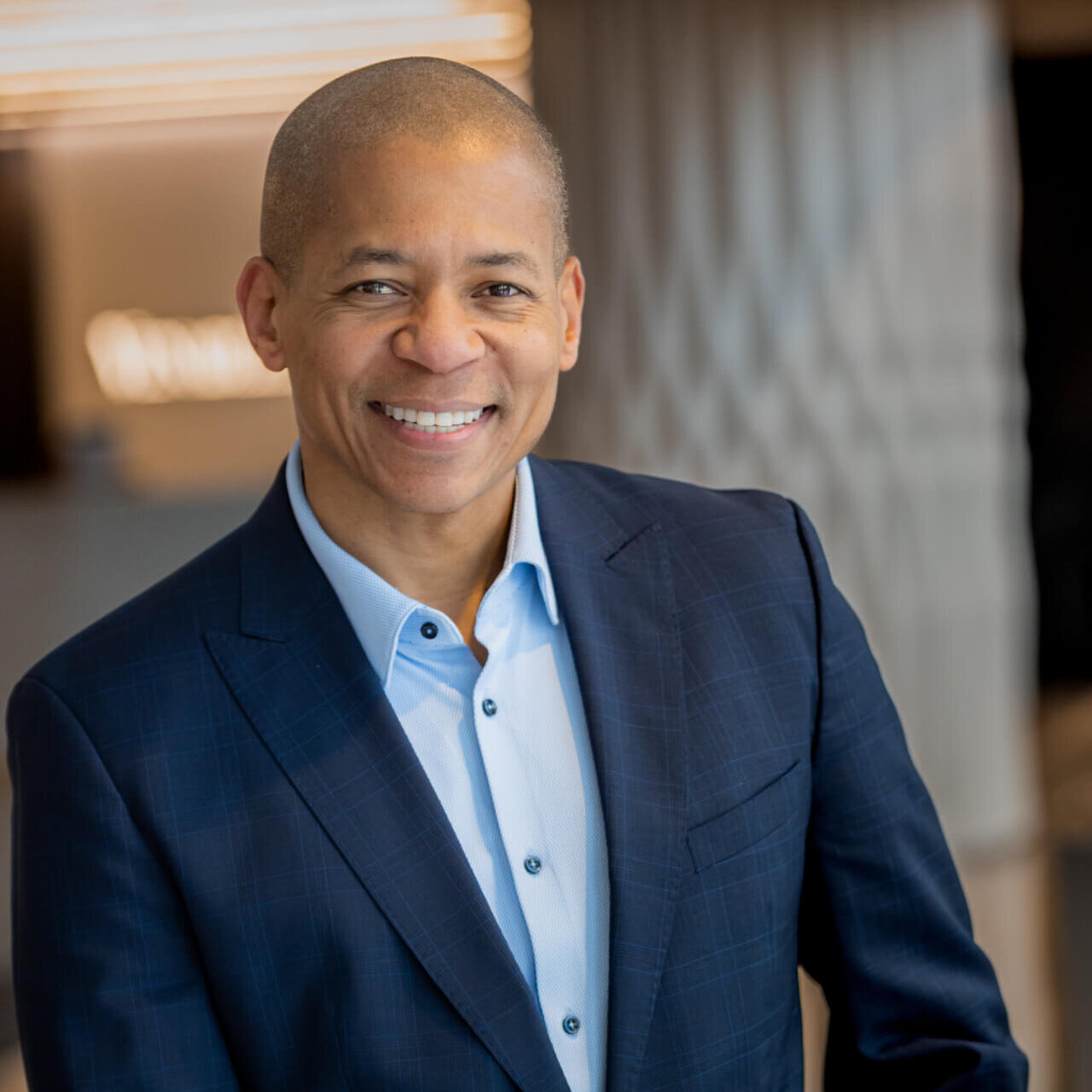
Damon Davis, MBA
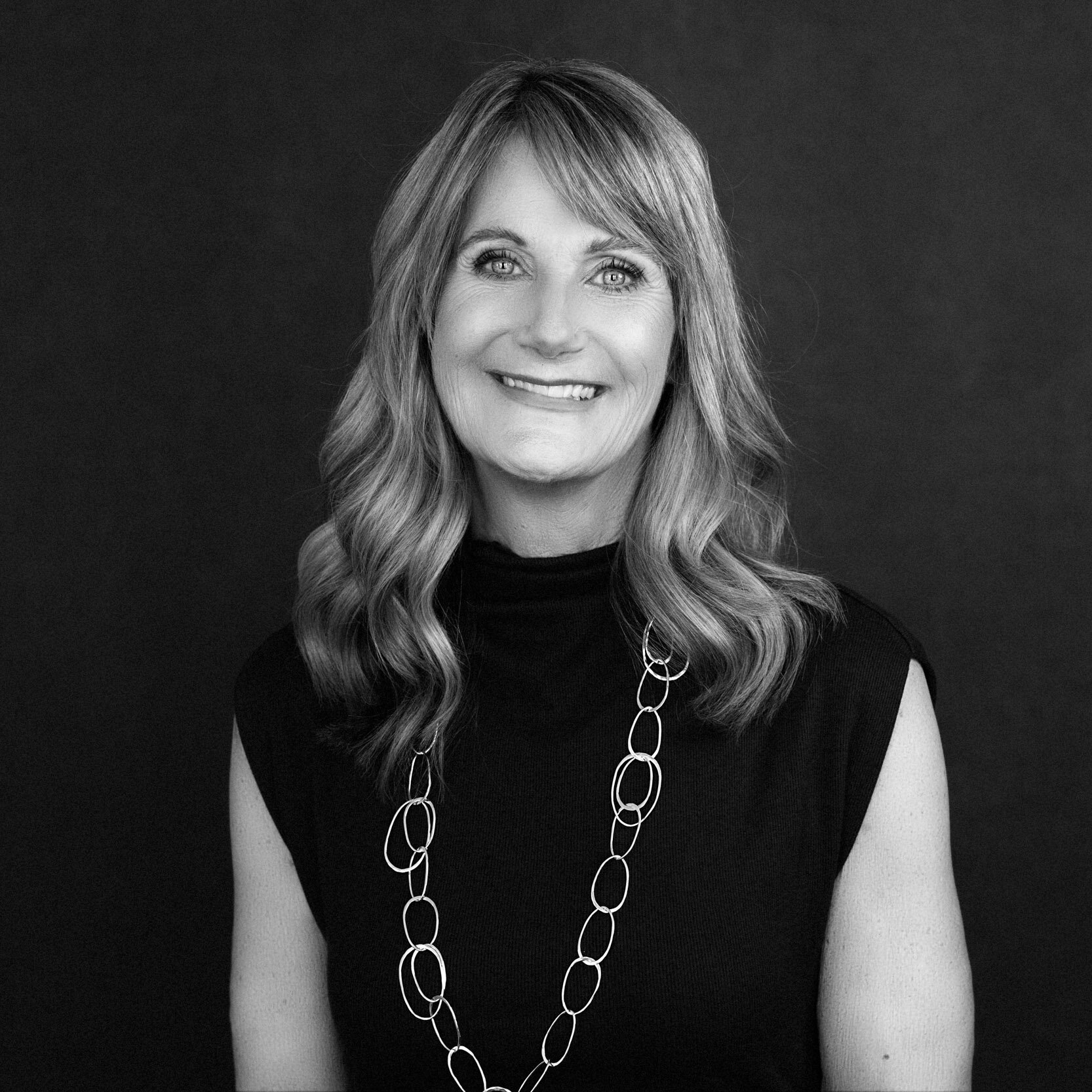
Deven McGraw, J.D., MPH, LL.M.
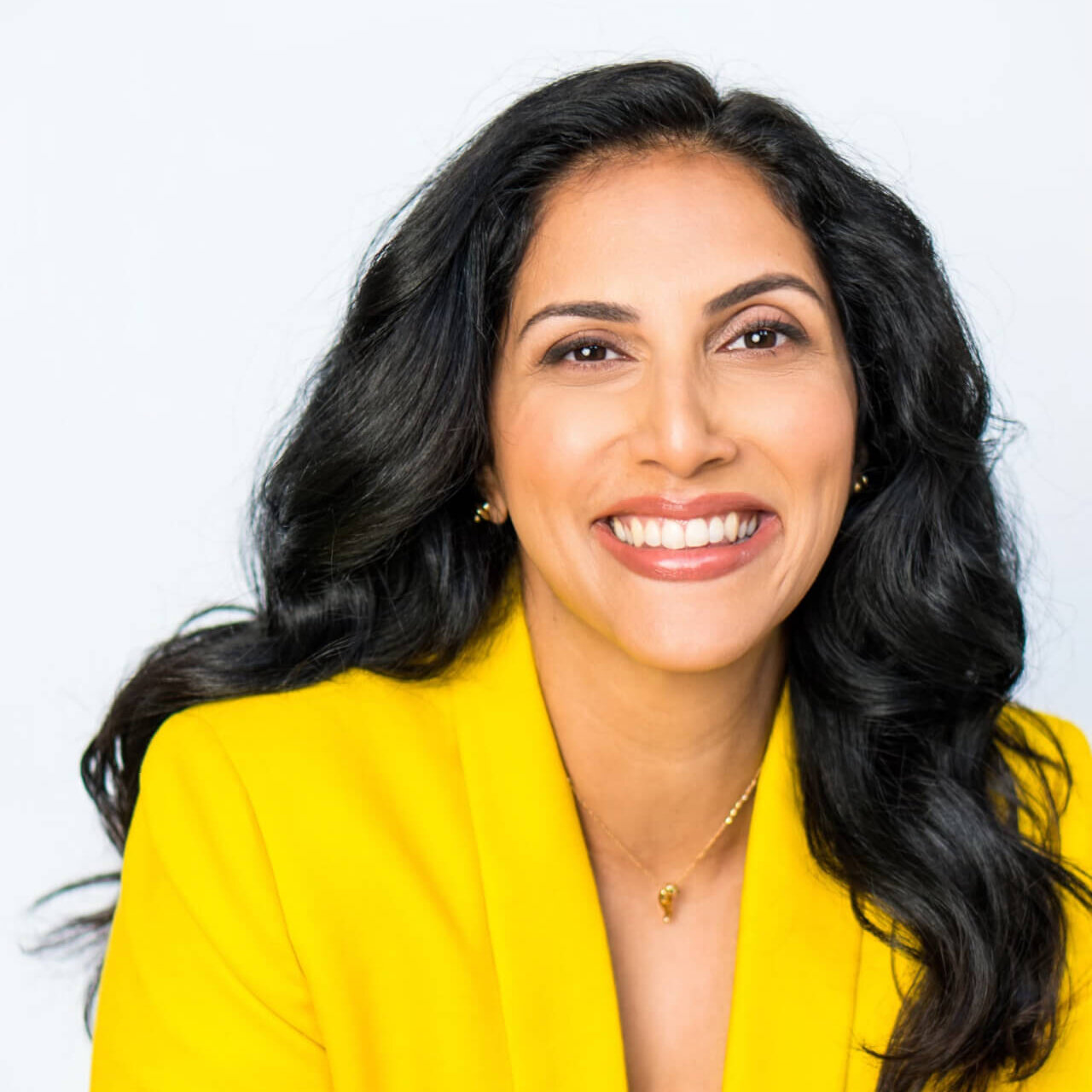
Geeta Nayyar, M.D., MBA
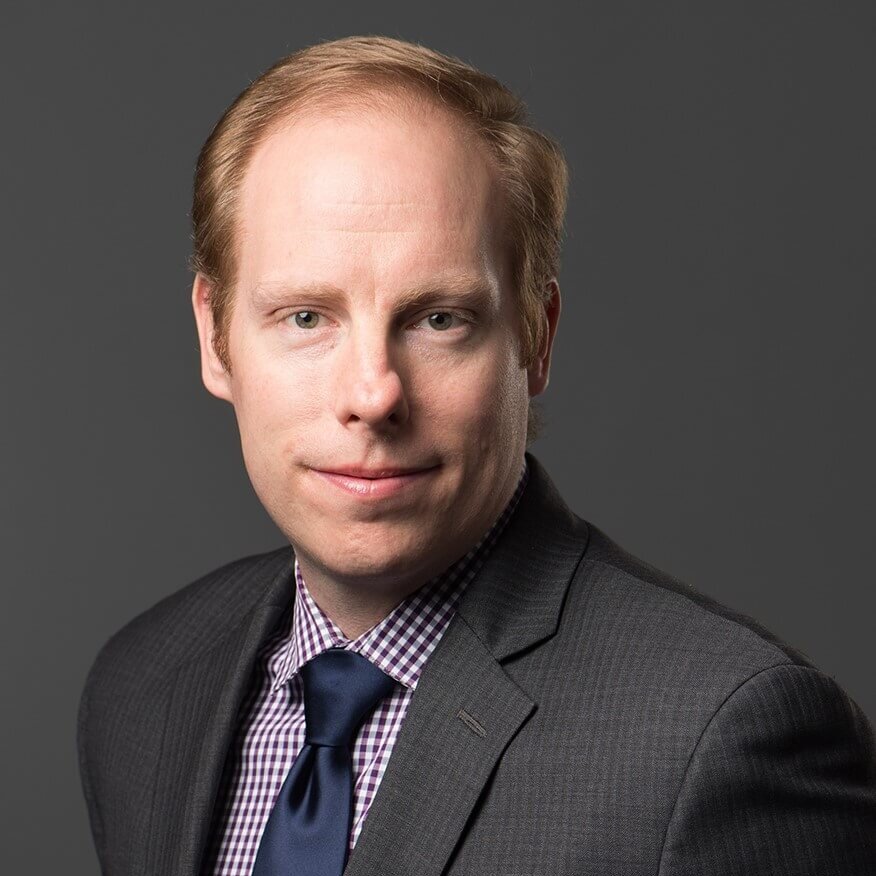
Jeff Smith, MPP
Transcript
Speaker 1
Good afternoon, everyone. Thanks for staying and I’m so glad you’re still here. This has been an amazing day of discussions and I’m looking forward to one more with some incredible panelists. So thank you for being here. Once again, I’m Damon Davis. I host Discovery Diaries with Damon Davis, and I’ll be your moderator today. This panel is really focused on trying to give some implementation ideas to policymakers. We want to ensure there is an effective balance in AI implementation. We want to make sure that it is sort of safely promoted, that privacy considerations are taken into account. And that there’s also a diversity of data that is going to be representative of basically what this room and what America looks like. So we’ve got some special panelists here today that I’m going to introduce, first of all, to my immediate right, Devin McGraw, chief regulatory and Privacy Officer at Citizen Health. Next, Doctor G Geeta Nayyar, chief medical officer, technologist and Wall Street Journal bestselling author of Dead Wrong. I hope you got that book when you walked in here today. And then finally, Jeff Smith, deputy director and of certification and testing at the Division of the Certification and Testing Division at the Office of the National Coordinator for Health Information Technology or ONC at the US Department of Health and Human Services. So with that established, I’d love for us to dive in. One of the big challenges that we have is obviously the potential for upside and downside to AI and Devin. I’m going to start with you in terms of ensuring that AI solutions are implemented in. Systems, how do we make sure that they have access to high quality data, but also a diversity of data?
Speaker 2
Yeah, I mean, we’ve talked a lot today about how AI is not going to serve us very well. If the data that feeds into AI algorithms is not diverse and not representative of the US population, which is very diverse. But of course, part of the problem is, is that that our health, you know, today our healthcare data is largely siloed. And even if we’re talking about where we typically get data from places where patients get service, oftentimes even those data sets themselves are only representative of the population that tend to use that facility. You think about all the interoperability initiatives that that we have funded as a country and that Jeff is helping. Put into place that are designed to get data flowing more so that wherever patients travel, they’re, you know, they’re all their data is there. Nevertheless, if if if that non representativeness of data does not get easily solved. Especially if the patients that we really need to make sure are in the data sets aren’t even getting care to begin with, so no data is being being generated about them. So it’s a big challenge. You know I I want to give an example of the federal all of US research program. Which deliberately went to over sample underserved populations. And what we’re finding is that some of the publications that have resulted. From that are really showing us what what can happen when you are very intentional about building a data set that is in fact representative, and I’m going to read out some numbers because I don’t memorize them. It’s not high level math, I promise. But part of the data that’s being collected all from from volunteers who are consented. Is genetic data. So there’s genetic data. People give consent for their electronic medical records to be part of this. They also get measured and weighed, and some of them have medical device data going into it. So typically genomic data, research data sets are 90% of European descent populations. In the all of us research program, where nearly 46% of the participants self identify as non European race or ethnicities they are they have found more than 275 million new genetic variants. This database has not been in place for all that long. And researchers are are looking at this very diverse data and coming up with data that is brand new, that isn’t present anywhere else and it just I think it underscores. The important you know it, it underscores a lot of things really it’s it’s it’s if we don’t if we’re not, but but mostly I think it’s if we’re not intentional about making sure that we are getting data from populations that we are not serving well today. We will continue not to serve them. We will continue to build algorithms that don’t respond to them we will. Exacerbate disparities in care instead of making them better, and I don’t think we’ve done as much work to talk about. What does it take to be really intentional, intentional about reaching populations that are missing in our data?
Speaker 1
I’m so glad that you brought out that all of us data. Yeah, I mean, that’s the intentionality of the project. Had an amazing spirit behind it. But just to hear that, it’s not even fully implemented. And already this amazing array of insights is coming out shows huge promise for what this project, let alone all of the hopefully. Later, deeper, more interconnected projects will will come out with. As well, I guess the the challenge I I’ve known you from my days at ONC and you’ve always been sort of a privacy queen that I’ve I’ve we’ve turned to and and so therein lies the next question is within is the challenge with privacy regulations. AI Electronic health records put pressure on privacy considerations. And now we’re talking about AI. There’s a huge interconnectivity between all of this data, these systems. What is the new pressure that AI puts on privacy considerations and and how could any policymaker sort of address these issues?
Speaker 2
Yeah. Well, it. I mean, if you think about. About what had to be done in the all of us program just by way of example, to make sure that people who are in underserved populations, who tend generally not to trust the healthcare system or their collection of data to actually have them be willing to have their data be collected and be part of this database that was going to be made. Accessible to all kinds of researchers without them necessarily consenting for each and every research opportunity. That’s a big leap of faith, and takes a ton of work to design something where people feel comfortable. You know it’s only deidentified data they did and they took another step to get certificates of confidentiality so that that what that means is that there is very limited ability to use that data in a context that could be harmful to that person like like criminal cases for example. So, you know really went. Above and beyond to try to protect that data. So what does that mean to, you know, for the ordinary healthcare system that’s that’s working on AI or vendors working on AI, who may not necessarily be able to access certificates of confidentiality. What kind of things do we need to do to the rules that we already have in place, you know, and the and some of the comments throughout the day struck me. I you know it. That there is, I think, on the part of patients and understandably so, a great deal of skepticism. About the use of their data in AI, but I think there are lots of reasons for that. One is that you know a lot of times with new and shiny things. In healthcare they don’t get built necessarily, they get, they get built, not necessarily with the needs of patients in mind, but the needs of the rest of the system in mind. And you know, we have, we do have a set of rules around how certain types of health data in the healthcare system, doctors, data from most doctors. Data from hospitals data from health plans gets governed by HIPAA. If it’s here in this country. But there’s a whole lot of data that sits, that’s very health relevant that sits outside of that framework that isn’t really governed by the same set of comprehensive rules. So we don’t have a good kind of baseline to build on in terms of how we protect that data. At the same time, how much more do we need to do? Even if we think about just the HIPAA data sources, it’s a lot of really sensitive data. It’s a lot of really important data that could be extraordinarily helpful in building AI algorithms. You know, we have some rules. Frankly, that are pretty good and have stood the test of time to some degree in how do you know when can you use identifiable data to treat patients? When can you use it to get paid for care? When can you do use it to do quality assurance for public health and it doesn’t necessarily mean that we have to say ohh because it’s AI. Patient consent is needed. But there might be a need for us to do more, for example with how we regulate deidentified data, which is frankly what’s going to feed most of these algorithms. And today we don’t regulate it at all. Nothing. If you if if you have A, you know, even an entity covered under HIPAA. That once they’ve deidentified their data in accordance with HIPAA methodologies, which only means that there’s a very low risk of reidentification, not that there’s zero risk of reidentification, it falls out of regulation. Altogether, we don’t even actually even have penalties for when people reidentify data, because it’s it. It was released into the universe with, you know, with no rules on it. And so it it gets monetized on a regular basis, even outside of the considerations of AI. And it just builds. Levels of mistrust, I think. I think when individuals don’t even really have transparency around how their data are are used, particularly in the deidentified context, and there aren’t very many collection limitations in HIPAA. So if an entity like let’s say I work for an academic Medical Center and I’m developing AI and it occurs to me that ohh just the data and the medical records that I have here is not going to be sufficient for this AI. I’m going to go to a data broker and scoop up a whole bunch of data on how people use social media and environmental data and income level data from my populations. I’m going to feed all that into my AI algorithm. There’s no limit under HIPAA to collecting that data. Some of that data, frankly, won’t be considered to be Phi because it’s building an algorithm. That, and it’s not necessarily being used to treat people as of yet. So there’s a lot of questions and and of course, again it can be monetized in ways that a lot that isn’t always transparent to folks so. So I think we’ve always had these issues with the shortcomings of our privacy rule situation. What AI has done is just exacerbate it. It did didn’t create these problems. You won’t find the words artificial intelligence in the in any privacy rule other than maybe some new stuff that might have popped up from state legislature. So there’s a bit of a long winded answer, but I think it’s a kind of a complicated scenario where this.Is innovation that we need to happen, but we need to have it happen in the right way. So how do you build the right set of protections around the data that still enable it to be used for the right purposes but but doesn’t necessarily deepen the mistrust that people already have, that many people already have in the way that we use their data and particularly again populations that we have not served well over time.
Speaker 1
Yeah, not served well, will unfortunately continue to be underserved if we are not careful. And you’ve mentioned a couple of things that I want to bring, Doctor. G in for so Geeta. I know you focus a lot on Health Equity and you’ve been sort of an observer of technology implementations over years, electronic health records, social media, some many of the things that Devin has already alluded to. I’d love to hear from you more about sort of our lessons learned from past technology implementations and how we can use them to be considerate. Of our current healthcare implementation of AI, what are the lessons learned from the past that we need to bring forward and and drop right now into our current situation?
Speaker 4
First of all thanks so much for having me and the team has done a wonderful job and thank you all of you for still being here, appreciate it. Look, as far as the lessons learned, I don’t know if. We’ve. Learned. Them yet, but the lessons we should have learned, I feel like you have to change the question, right? The lessons we should have learned is number one, the problems we have in healthcare it they’re not about technology, right? And I think, Devin, you made a great point in saying that, right. The problems we have. In healthcare, whether it’s Health Equity, whether it’s a burnt out workforce, whether the fact that technology was supposed to help our workforce and actually ended up burdening our workforce, and now they’re incredibly turned off to anything technology, even if. We do neat and cool exciting things with generative AI. Half the battle will be convincing the staff that it is actually going to work, right? I think the first thing we have to remember is the excitement we have around generative AI, myself included. As a technologist is is limitless, but as a physician I’m slightly skeptical because we have to make sure that this this era of innovation has to be done with our clinicians, with our doctors, with our nurses, with the care team. It cannot be done to them. Which is the way the EHR has really been done to date, right? We also have to be mindful that similar to the EHR, while we may have solved 3 problems, we created 5 new ones. Right. And this time, we can’t afford to do that because we have a serious workforce shortage. We have a serious burnout problems. One in five healthcare workers are leaving the industry since 2020. And if you’re in rural America, you’re even worse off. You have less than 10% of physicians even working in rural areas, and one in five Americans are in rural areas. So this is not a small number, and it’s often also lost when we talk about HealthEquity, when we think about the underserved rural America is at the top of that list. And so I think there’s limitless. Potential. But we have to remember what is the problem we’re trying to solve today. And when you talk to technologists, by the way, they are of course the most excited and they will tell you that, OK, we’ve got a workforce shortage. We have a burnout issue. We can solve that. We can solve that with AI. We’re just going to replace all the doctors and nurses and boom, we we got the solution, right. How many, How many people believe That’s the solution. Right. We know that what drives consumers in to see the doctor is the relationship. We know that 92% of all Americans still trust their physician. They may not trust the system. They may not trust the insurance company. They may not trust the pharma company, but they trust their. Doctor. Right. That is what makes them make the appointment fundamentally. So if we are going to try to get in between that relationship, it is the wrong problem to solve. Now, if we could instead focus on that relationship, focus on that trusted relationship, focus on the humanity that is ever present in healthcare and instead de-burden the care team take take prior off off of their table. Take documentation off the table, focus on drug development, diagnostics, therapeutics so that doctors, nurses. Have more time with their patients, more time to look them in the eye and less of the friction which is largely in the back end right largely in the back end. No, it’s not sexy. It’s not R2D2 seeing patients. But it could save us billions of dollars and a happy doctor is a happy patient. You cannot have a burnt out workforce and expect to have a good consumer experience. We often talk about patient experience and consumer experience. We rarely talk about the physician experience and the care team experience. We cannot do that. This era of technology, this is our opportunity to get it. Right. This is our opportunity to take the time reflect to ensure that we have the clinical leadership from the beginning and that we’re truly pointing it at the low hanging fruit. not the R2D2 in the white coat, not the vision.
Speaker 1
Yeah, I love what you said there too, about the fact that Healthcare is inherently a human focused entity, right? We’re not healing cars. These are people. This is real relationships and we have to connect with each other and I love that you’ve sort of highlighted the fact that there’s so much that can be done with the technology towards the back end. That can be instrumental in making the front end the human interaction really, really meaningful. One of the things that I know you focused on over the course of your career is HealthEquity. And it’s been laced throughout multiple conversations here. But just chat a little bit about the challenge of AI. We we all have a vision for it, to eliminate health disparities, but there’s the potential for it to exacerbate them as well. Share a little bit of your thoughts on how AI may potentially be used to eliminate them, and what the danger of exacerbation of of disparities is.
Speaker 4
Well, look, I’m going to. I’m going to answer that in a little bit of a roundabout way. When we look at the gap in healthcare today and we look at where consumers are getting their health. Information it is not from their doctor. For the most part, it is not from any trusted resource. For the most part, most Americans do not have a doctor, and they are instead turning to TikTok. Right. It’s simply a reality. 59 million Americans turn to social media for information about their health and this market, right? Healthcare being on your proverbial wrist, your proverbial finger. The wearable market has exploded. It went from a $32 billion market in 2019 to a $54 billion market in 2023, which indicates the consumers. Willing to spend money out of pocket. Wants to measure everything from their sleep to their mood to their steps, even if they don’t know what it means, it makes them feel empowered. It makes them feel in control of their health. In a post pandemic world where we have been reminded health as well. So healthcare, we’ve left this huge gap open where people want health information, but we’re not meeting the moment, right? Doctors are too busy putting out fires. Health systems are too busy putting out fires. They’re not looking to see who’s causing them. And here’s what happens. You follow an influencer on TikTok and they tell you. Look, if you’re worried about breast cancer, you’re worried about getting old. Just buy this gene kit for 1099, and we’ll tell you if you got to worry about that. And if you do, we’re going to sell you this supplement for 1299, right? So what happens? You don’t get the mammogram. You don’t get the colonoscopy, you don’t even go to a doctor. Cause why would you? This person like knows you. You get to see everything about this. Person. So we think about equity and we think about myths and disinformation. The reality is that myths and disinformation is not new, been around since the Black plague right there was always a neighbor. There’s always somebody who had some ginger turmeric in the South Asian community that sells everything, right. But the difference is it’s getting worse. So miss and disinformation now is traveling six times faster than the facts because of the technology, because of social media, because of WhatsApp. So if we throw AI into the mix, which we are. It is only going to get worse and the healthcare system will only get more burden because by the time those consumers do come to us, it’s too late. They have the cancer and it’s metastatic, right? And it gets more expensive, but it really goes to the trust issue because the reason communities, particularly black and brown. Communities look to other sources is there’s a lack of trust in the profession of medicine in the healthcare system. And so it’s really incumbent on us to win that trust back. But to think differently and the the prime point that I’m trying to make, is that the bad habits we have today and Devin, you covered this really beautifully. The bad habits we have today around data silos, interoperability, gender bias, racial bias, cultural bias, all of those things still exist. AI will only compound our bad habits. We still have to do the hard work of fixing our habits. Missing disinformation is simply. One example of that. And we have to remember that generative AI is only as good as the data we give it. I can’t tell you what the HR has done. If you and I see John White was in the back there, any Doctor Who reads a note today, it is now for those who were around during the Encyclopedia Britannica days, it is now a chapter in the Encyclopedia Britannica. When our notes used to be about two sentences, if you were a surgeon and maybe four if you were an internist. The note doesn’t make any sense. It doesn’t make sense to a doctor or human doctor, so to put generative AI on bad data. It’s very risky, it’s very risky, and halfway when you read through, no, he turns into she half the time, right. So we have to remember all of these bad habits. Actually, a lot of them came from the tech that we implemented erroneously. So I am a big believer in AI and what it can do, but we. As healthcare leaders still have to do the hard work. Interoperability will not be solved by AI. Will we be able to Jimmy rig it and go around it? Faster and better, yeah. But ultimately the issues around data still exist.
Speaker 1
Exactly. I think you said some things that triggered for me, one you know as people of color and folks who are in communities of color and traditionally sometimes have less access to the healthcare system. We’ve talked before about how if the data is pulling from those who have had access to the healthcare system, those who have not had access will be left out of the data and therefore will not be part of the algorithms that is trained on. Secondarily, I think about, you know, the White House has an initiative on research in Women’s Health. Women have traditionally been underrepresented in healthcare research. What does that mean for the data that is going to be utilized for AI algorithms where women have been underrepresented in a lot of research, too? So there’s a lot of different ways to slice this, I know. Stephanie was on the prior panel. I spoke with her upstairs about the under representation of people with disabilities and rare diseases and things like that. There’s all these slices of humanity that are not currently well represented, that when AI is put on top of some data. There’s going to be some challenges in pulling meaningful insights that are accurate and effective for the healthcare providers to to delve into. Jeff, I want to turn to you for a moment. And first, maybe you can tell us about this morning’s announcement. Your boss went from having a pretty simple title in the office of the National Coordinator for Health IT to probably. The longest title in government just say a little word or two about the change in your office, and then I’d love to hear about some policy regulations around AI.
Speaker 5
Yeah, sure. So yeah, so we took a a very large mouthful, which is the national coordinator for health information technology and decided to add some more letters to that we are. Now the assistant Secretary for technology policy Slash office of the National Coordinator for Health Information Technology, I had to practice that several times on. The way over but. I got it essentially.
Speaker 4
Three times faster, three times faster.
Speaker 1
And you know in government they like to pronounce an acronym. So I can’t wait to hear how you actually pronounce those 14 letters.
Speaker 5
Yeah, AST P/ON C you know me.
Speaker 1
Well, blade.
Speaker 5
Yeah. Yeah. So, you know, I think just real quick, this is actually I I think a really important development, not only for ONC, which has been the coordinator of of HHS IT policy for over a decade now, but also, you know, essentially what this does is it elevates the office within the department. And we’re also going to be bringing in the office of the Chief AI Officer as well as the Office of the Chief Data Officer. And we’re going to be reinvigorating the concept of a chief technology officer. So we are really kind of consolidating all health data, technology policy and kind of HHS deployment within under one roof. And I think that’s going to be really very positive just in terms of truly trying to be coordinated across the department. So anyway, that that happened at 8:45 this morning in the public inspection desk. And I know we’ve got people out. There that know what that means. So.
Speaker 1
Talk a little bit about the the current regulatory environment then what it what is, what was formerly ONC and HHS working on what and what other other channels where regulatory policy is is overseeing and and supporting AI’s development.
Speaker 5
Sure. So you know to try and bring some of what my my fellow panelists have brought to bear to this I you know, we hear that burnout is a problem. We hear that disparities are a problem. We know that privacy is a concern. We know that within the. Data representativeness is a concern. We know that artificial intelligence has demonstrably created bias and racial disparities, and. When we were first charged at ONC, looking at the the general space of AI back in, I believe it was April of 2021. We were, we were told we need to fix the problem of of racial disparity in AI. And when we looked into it, we saw a heck of a lot of different problems. Racial disparities being one of them, and and so. It, all of this actually makes a lot of sense, and you know, I think it’s it’s actually really difficult for any single federal agency to kind of look at the landscape of problems and say we’re going to try and fix all of it. But that’s actually what we tried to do at ONC, and so our our guiding principle was how do we optimize the use the widespread use of high quality predictive algorithms and now generative AI and we we determined well in order to do that, the AI has to be demonstrably fair. Appropriate, valid, effective and safe. If we can figure out how to bring transparency into the performance of predictive models. Then we can start to optimize the use of this technology so that clinicians trust it so that patients know it exists and so that it doesn’t perpetuate bias that has been in the data cooked in the data, either because the because data are missing or because data are incorrect. And so it was really with that vantage point that ONC in specific said, we are going to. require a certain segment of the market to ensure that they provide transparency on the performance and the quality of the predictive models that they supply to their customers. Now because ONC regulates electronic health records, our authorities only extend so far our authorities extend to the certified EHR companies that exist, by the way they exist in 90%. 96% of hospitals, 80% of office based clinicians. So we have a good footprint across those developers. There’s, I would say 20-30, forty maybe more predictive technologies that will and generative technologies that will be subject to our requirements, but it only represents a fraction of of the overall. And so when you start to think about the big broad picture of regulation in the United States as it relates to health, AI, a lot of people talk about a patchwork. Those who want to be a little bit. More friendly talk about. A quilt. I’m going to talk about a regulatory mosaic because we’re very purposefully trying to build a nuanced and detailed picture of regulation in the United States when it comes. To health AI. And what that means is we depend on the FDA to a certain degree to work within their authorities. They’ve approved or sorry authorized. There’s a difference inside of the government. They’ve authorized more than 800 almost 900 AIML driven medical devices over 150 in the last year and they’re looking at safety and effectiveness. Right. So they are the gatekeepers, ONC. Has come in. We will be regulating some of the same technology, but a lot of different technology, different technology than the FDA cares about or different technology than the FDA is focusing on, I should say. And we’re going to be regulating for transparency. So we’re we’re trying to enable users to make determinations whether or not. The predictive algorithm, or generative AI that they’re using is fair, appropriate, valid, effective and safe, and we’ve created this kind of model nutrition label concept and then I know Jenny MA from OCR was up here before lunch and she was talking about focusing on, not necessarily the development, but the use of AI. And so I think what you’re starting to see is a coordination and an effort that’s been very deliberate. And I can say it’s been very deliberate because I’ve been on and my colleagues have been on phone calls with with the OCR and with FDA numerous times trying to figure out how do we work within the bounds of our existing authority to really start to make an impact. And and and you know and and again I think just to put it one more fine cap just given the flavor of the conversation. So far, another piece of our requirements would ensure that users of electronic health records have information on whether or not certain data elements salient to HealthEquity like race, ethnicity, gender, sexual orientation, social determinants of health, health status. If these data elements are used as part of inputs. To predictive generative or even just rules based decision support, users are going to have are going to know that. And guess what? When we were talking about EGFR and when we’re talking about all of those risk calculators, starting actually even last week Med Calc came out with a new policy that said they’re going to flag every time that race is used in one of their numerous calculators. And and again, you know, I.
Speaker 1
For evaluative purposes.
Speaker 5
It’s it’s just so the users know. So that they know. That this very simple rules based calculator uses race and and and you know flags that and you know again I think the the individual from arc AHRQ you know they did a lot of work to try and figure out is the use of race appropriate or inappropriate and what they came up with is it depends. It can be bias. Promoting it could be bias. Reducing it could be bias neutral. And and so I think the first piece of it and this is where we focused all our energies was on transparency. We have to be able to understand if it’s there and then if it is there, we can make a determination as to whether or not it should be there or how it should be applied. So it’s a, it’s a fairly you know it it’s a very nuanced space, but the. Government is trying.
Speaker 1
Yeah it’s tough and so I hope you guys are getting ready for your questions. We’re almost time ready for you, so get your hands ready to go up. One of the things though that triggered for me when you were talking Jeff is in the use of race. We tend to talk about them in monolithic terms, right? There’s only a couple of boxes that you can check, but for example, my wife is born of a white Canadian woman and A and a black Canadian guy, right? So how do you, how does she check? A box. And therefore, how is race utilized in her medical record? Right. These are the challenges that we live with, let alone you can’t just say Asian is that East Asian is out South Pacific. Like how does it work so very, very challenging in terms of race. Final question for you. There’s got to be some sort of places of urgency where AI really needs. Immediate attention. What are some of the hotspots that you see are absolute necessities for us to focus on, right in the immediate term?
Speaker 5
Yeah. So probably somewhat unsurprising to you, Devin. The place that we need a lot of attention is in that health tech space that exists beyond HIPAA exist beyond OCR, exists beyond FDA exists beyond ONC. And see, you know, I think the other day we were trying to sit down and and, you know, literally look at OK, of the 882 medical devices that have been cleared or authorized by the FDA, how many of those are electronic health record developers, super small fraction, very, very small. OK, so we’re covering different ground. We really are. What about all of the technology that exists within a hospital or healthcare system or just outside of it and their consumer facing that are not Regulated and even within a healthcare system, I mean, there’s a lot of technology, a lot of technology companies. I think a lot of really good ones, probably a lot of bad ones that are not going to be certified by ONC and maybe they may be doing something pre processing, they may be doing something that you know technically exists outside of the healthcare system, but then gets brought into the healthcare system. Through one way or another, and the FDA may not know about it or it gets self developed and that’s still a space. You know, the hospitals. I think are many of the major hospitals are really stepping up to the plate and starting to get much more serious in terms of their internal development and their internal validation and testing of stuff that they themselves self develop. But the vast majority of users out there who get their technology from developers from third parties. They’re buying it. They’re putting it in and that’s it. They’re not validating it. They’re not. They don’t have comprehensive monitoring over it. So, you know, I do think that this space where current OC, FDA. OCR authorities and there’s others out there. CMS could be playing a role. Maybe they will in the future, but that’s that space that it exists beyond the immediate kind of healthcare delivery is really important.