Leading the Charge: Executive Insights on AI’s Impact
1:40 pm
This executive insights panel will examine current uses and applications of AI, share innovative ideas and advancements that are driving significant change in health care, and provide valuable considerations for policymakers aiming to support and regulate this rapidly evolving field. Join us for an enlightening discussion on the transformative potential of AI and the strategies needed to ensure its successful implementation in health care.
Speakers
- Moderator
- John Whyte, M.D., Chief Medical Officer, WebMD
- Panelist
- Ian Blunt, M.Sc., Vice President Advanced Analytics, Highmark Health
- Jesse Ehrenfeld, M.D., MPH, Immediate Past President, American Medical Association
- Vincent Liu, M.D., M.Sc., Chief Data Officer, The Permanente Medical Group, Kaiser Permanente
- Danielle Lloyd, MPH, Senior Vice President, Private Market Innovations & Quality Initiatives, America’s Health Insurance Plans
Summit Details
This panel is part of a larger summit event.
July 25, 2024
Speakers
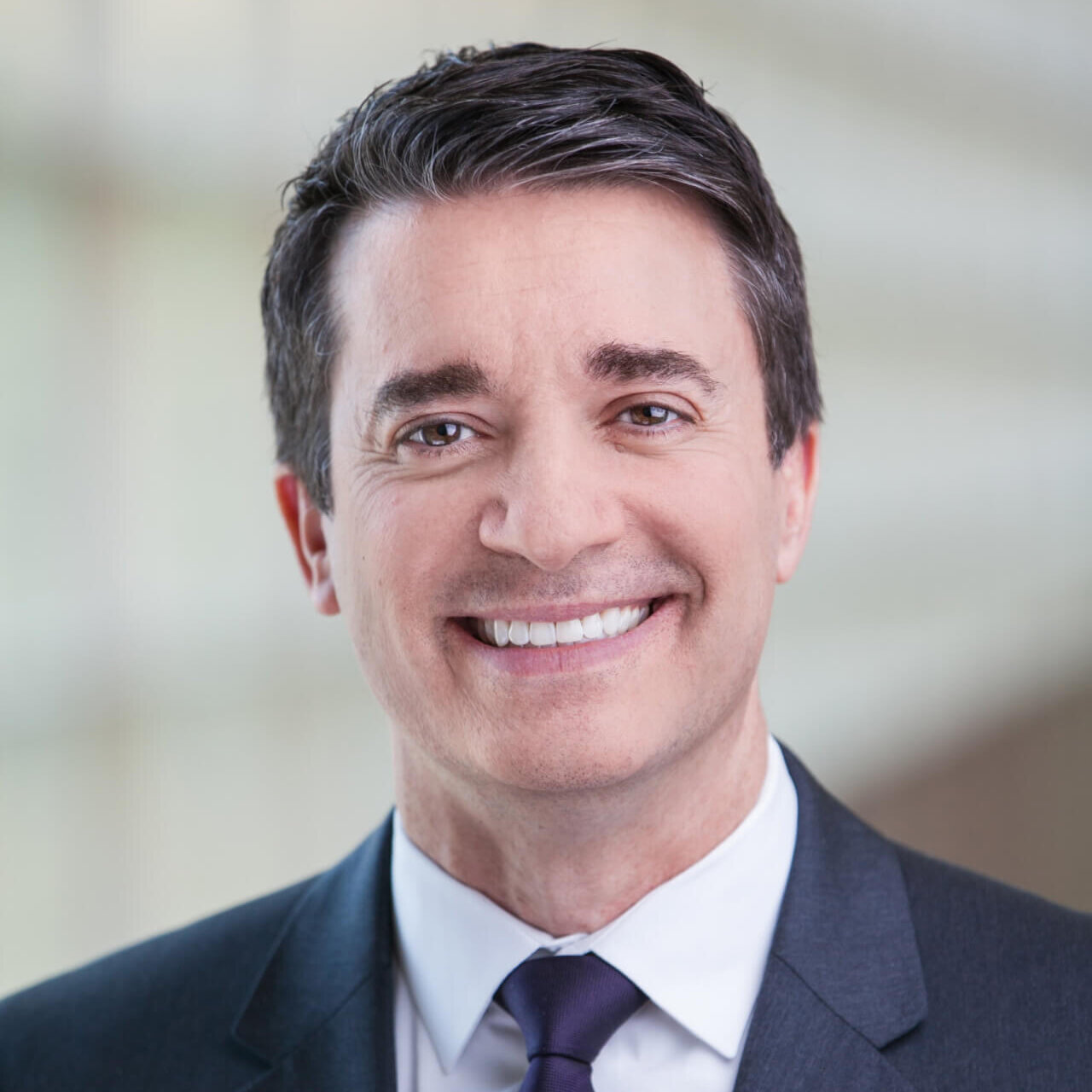
John Whyte, M.D.
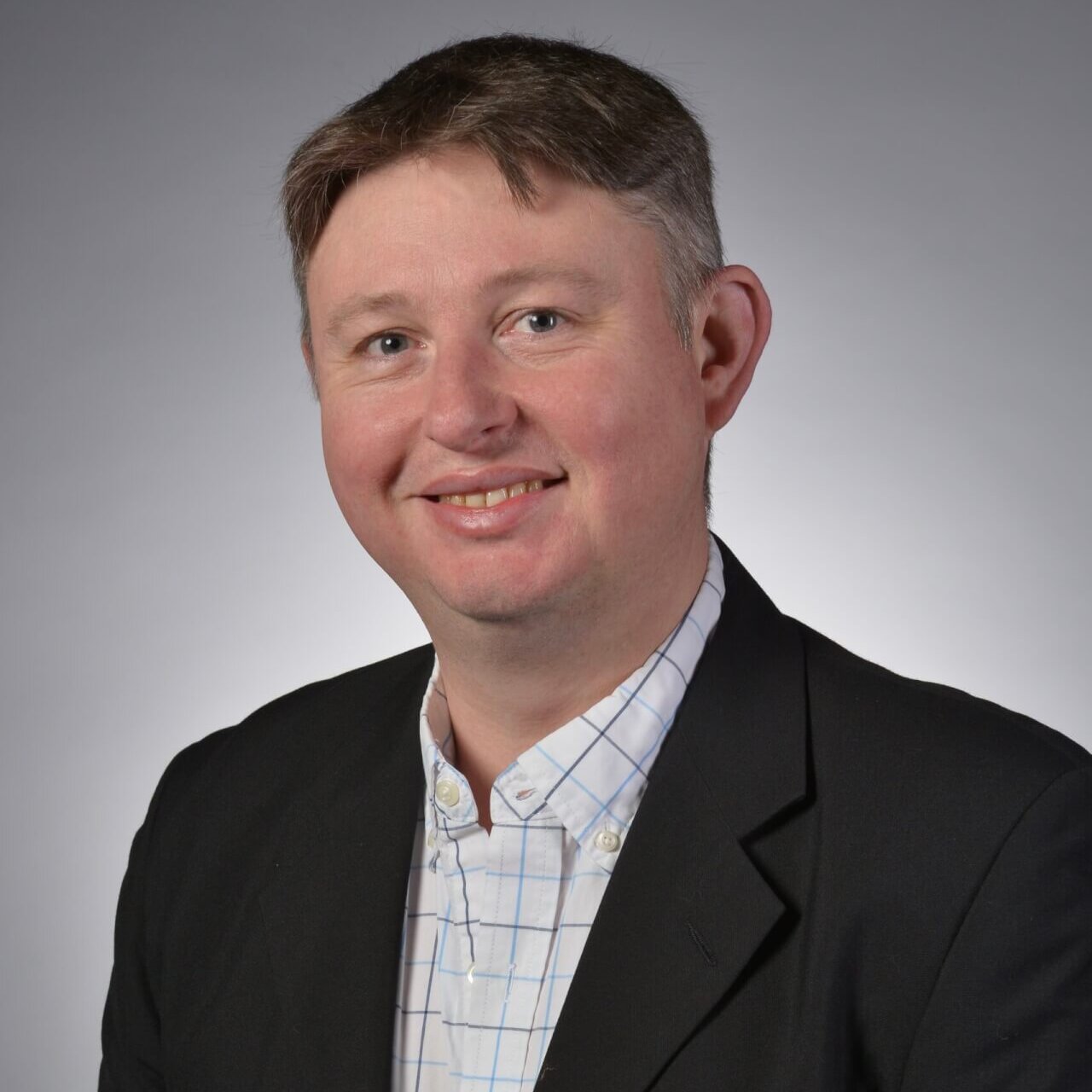
Ian Blunt, M.Sc.
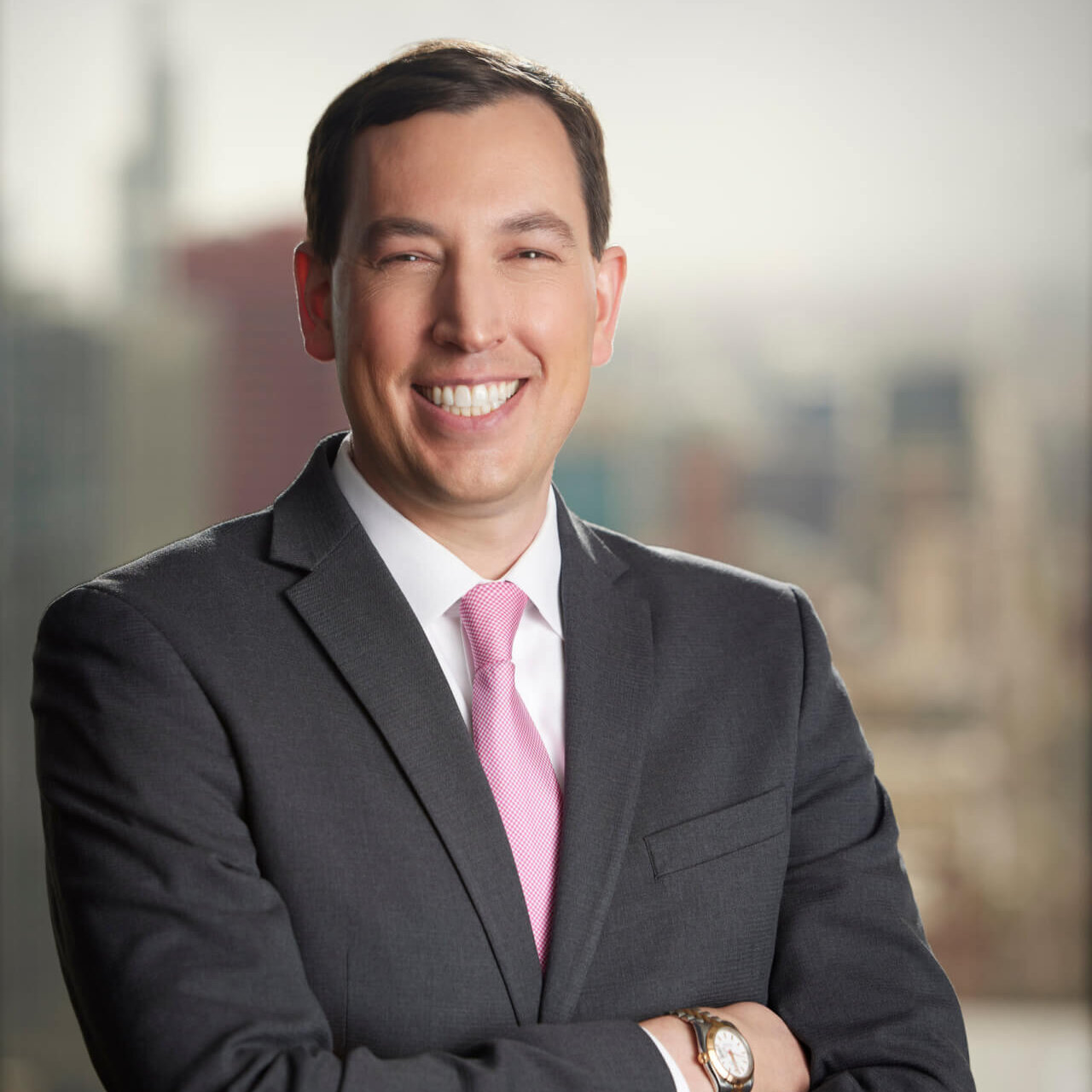
Jesse Ehrenfeld, M.D., MPH
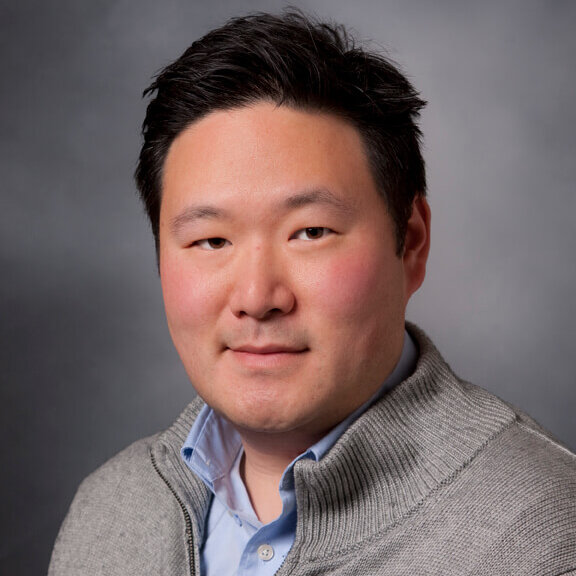
Vincent Liu, M.D., M.Sc.
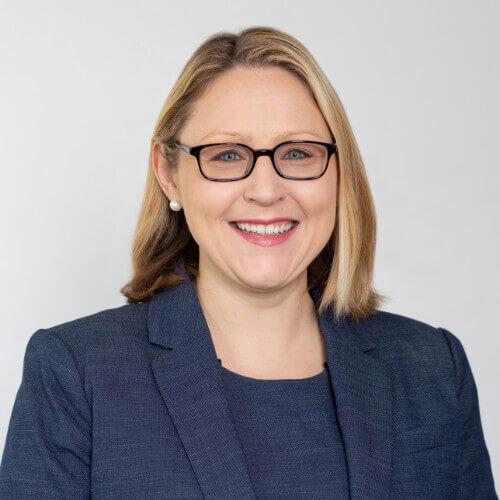
Danielle Lloyd, MPH
Transcript
Speaker 1
Well, welcome everyone. I I think we’re going to have a robust discussion about AI and I’m delighted to be joined by three experts seated at the far end is Doctor Jesse Ehrenfeld. He is the immediate past president of the American Medical Association and we have Ian Blunt, who is the Vice President of advanced. Analytics at Highmark Health. And next to me is Danielle Lloyd, the senior vice president of private market innovation and quality initiatives at American Health Insurance plans. AHIP. Panelists, thanks for joining me. Now we we do have some prepared questions and they don’t know I have some extra questions that I’ve thrown in. But you know, we all like to be positive. That’s how we should start off any discussion, even though we’re in Washington, let’s be positive. So I’m going to start off and ask each of you and we’ll start with Danielle. Ladies first. What are you most excited about? When you talk about AI and healthcare, what excites you?
Speaker 2
So I appreciate starting off with the positive and Speaking of which, thank you for having me here today. You know, I think what we the ways in which we were using AI so far is really just the tip of the iceberg. There are so many different, exciting areas. To come that we can envision, you know, I don’t think anybody gets up here and says I’m super jazzed about administrative simplification. Right. But it’s, you know, I think it’s something that shouldn’t be discounted because to the extent that it saves money, that’s money back into consumers pockets the extent that it it it saves time. You know, that’s more time in Jesse’s day. He gets to spend, you know, with his family instead of doing administrative work, but of course I think why we’re sort of all here is, is the potential for it to transform care sort of where and how you get care and better outcomes. I will say on a personal note, I have several autoimmune diseases and probably a few yet to be diagnosed and it’s really frustrating when you go to the doctors and they’re like well. We know it’s not going to kill you, but we don’t know what it is or why it is and we can’t really help you, right? So I I think like this notion of being able to take data not just within the US but potentially internationally and bring that together and be able to do. You know, trend analysis and pattern recognition and things like that so that we can find new diagnosis and new treatments and the like. I think that’s part of it’s, it’s really great potential.
Speaker 3
Yeah. So I’m excited about the potential of AI in healthcare. I have been for 20 years, my entire career in healthcare. We’ve been saying AI is going to revolutionize it and quietly it has. There’s lots of innovations that come in over the decades. That have really improved the way the system is able to provide care for people, but of course for large the big leap forward for large language models took nearly two years ago. Now hugely significant, both in terms of the capabilities that it adds to the AI, what it can do, what it’s useful for, but also the way it kind of. Entered the mainstream and got everyone talking about it. So as a society, we’re going to have more and more engagement with what AI means. What AI is good for what? Maybe it’s not good for, and that’s going to lead to legislation as well. So this is a really important time to shape how we think about AI in general and particularly in healthcare. It’s got so much potential to do real good. Help. Of people, as Danielle said, find patterns in the data. That’s what it does. So we can give better treatments. I think also we know how stressed a lot of provider systems are. The ambient listening technology, massive potential to give back so much clinical time and you know reduce the risk of burnouts and help our providers systems continue to function effectively.
Speaker 4
Well, I would say that that potential across every aspect of healthcare, how we train physicians, nurses, healthcare practitioners, how we educate those in practice, how we deliver clinical care, how we simplify these back end operations that frustrate so many people in the healthcare delivery system. Today, that broad range of things is why I’m so excited about the potential of this technology and we so desperately need it right? 83 million Americans don’t have access to primary. Care today we could never open enough nursing schools, medical schools, training programs to solve that. If we’re going to deliver care the way we do today, right, we have to scale the capacity of our teams to make sure that people can access the care that they need when they need it, where they need it. The only way we can do that is through the use of these novel technologies and AI. You know, I I was in the operating room on Monday. I’m an anesthesiologist. I see patients still. And at the end of the day, when it was time for me to leave and go home and have dinner with my kids, there was a long list of patients. Waiting. As there is every night at 5:00, because we may have operating rooms, we may have surgical equipment, we may have medicines, but we don’t have nurses. We don’t have doctors, we don’t have enough team members to deliver the demand that exists each and every day. So the excitement that I see is to scale the capacity using these tools.
Speaker 1
So we have to have fair balance and just like every drug has risks and benefits, are there risks to AI and and Ian and you mentioned you said there are things that AI does well. There are things that AI may not do well. What are some of the risks that you see of utilizing AI in healthcare.
Speaker 3
Yeah. So the risks. There’s a quote from a famous statistician called George Box that I really like he said all models are wrong, but some are useful and that is the best description of AI that like anyone can come up with because what we’re saying is here’s a pattern weve spotted So here’s what I think is going to happen or what I think is required based on that pattern. Inherently there’s going to be outliers there. The the pattern doesn’t quite work, doesn’t hold true. Some weird reason. So every time we’re working with AI, we need to bring that on board and we need to do some really simple things that we can all do. If you’re an AI practitioner, we need to think about a responsible AI framework. So how do we know that the AI model we’ve built is good? Lots of the big cases we’ve seen where something’s not quite worked right. They were well-intentioned. No one was going out to be mean to predict an outcome. They had no business predicting. They just didn’t really think through the problem like they didn’t have an inclusive training data set or they didn’t test the results against the kind of right dimension to see that. The model was performing inequitably in some way and I think also there’s kind of two associated risks with AI as it becomes more and more prevalent. One is a kind of over reliance on AI, so if you remember when sat Navs came about in the early 2000s, there was these new stories about someone turned into a hedgerow because it said turn left. So they just did without thinking. Definitely our critical thinking skills, all of us as it relates to AI is important. Really interesting questions about liability. Which I’m sure get mentioned, but also there’s a kind of AI rejection as well. It came out of the computer the algorithm. It must be wrong and I’m going to spend extra time proving its wrong so I think the key for AI is to use it. You know, as a first draft mainly, but always have the kind of humility about does the result I’m getting make sense. Whether that’s a automated check or a human expert check.
Speaker 4
Yeah I mean there’s a long list of risks, you know, biased? Results inaccurate hallucinations. All the things that we hear about that we know are challenging with these models. I think the the biggest risk in my mind though is. That we implement systems and we erode the trust of patients, consumers and physicians and that would be, I think deeply damaging to what is possible in terms of the adoption of these innovations. And so to make sure that we maintain the trust of the people using these tools, we’ve got to have established standards and that could be through regulation, it could be through labeling depending on whether it’s regulated. Or not around things like transparency, right? I should never walk into an operating room, turn on a ventilator and not understand that the ventilator has an AI algorithm, is trying to modulate inflation pressures to optimize ventilation for my sleep patient. Right, the only way I can step in and correct an algorithm if something has gone horribly wrong. If there’s a sensor malfunction is if I know that there’s AI operating and I may never understand what it’s doing because explain ability is very challenging when you’ve got a million parameters and a huge model in deep learning and all these things, I get that, but we should demand transparency if we’re going to establish trust in these tools and systems as they proliferate.
Speaker 2
I think there’s also, you know, just sort of thinking like what questions I most frequently get asked by policymakers and such. Right, you know, I think one is there is sort of a fear slash misunderstanding of this is all autonomous AI run amok the computers. So, you know, going to take over everything for everybody, right? Totally. Not the case, you know, with all of these uses, you know and not To to steal Jesse’s Thunder on some of this. But you know, the AMA always says augmented intelligence instead of artificial intelligence.
Speaker 4
She said it first.
Speaker 2
Yeah. So, right, so you know that AI is meant to be a tool for humans, not a stand alone thing. Right. So I think there’s some education around that because we don’t want to see the policies just immediately try to regulate all of the risks and not allow the innovation and the benefits that come with it. And the second thing is around. Bias and in these models and sort of to what you said. Like there, there’s always going to be some bias or not. It’s what you do with. But but I think part of This is why we’ve been working with the Consumer Technology Association, among others around a a series of different standards, one of which is around bias mitigation. So I think part of that is making sure that everybody’s sort of aware it’s built into governance processes, it’s built into the standards. And that people are second guessing as much as they can and are ready for, you know, anything that might occur that they couldn’t second guess.
Speaker 1
Let’s get very practical to give the audience kind of where we are. Today. So what’s your impression of how we’re using AI right now in terms of work strength, because that’s really the delivery of care, it’s managing work streams. So what’s artificial intelligence doing today to help with that?
Speaker 2
Do you want to start with the clinical part? You. Want to start with the all? Right. I’ll start with the payer, so. So I.
Speaker 1
If those to get paid.
Speaker 2
Yeah, sure. It’s it’s a full circle. It’s a full circle. But you know the the payers have been using artificial intelligence for for quite a while and lots of different ways. But I’d say it’s, it’s what we hear from our Members is sort of infused through a lot of different business practices, right. So that there are some in. Identifying fraud and abuse and trying to speed up. The. Claims process in terms of their interaction with consumers of you know, for example. you know, identifying high risk patients who perhaps need some additional reach out, you know, of course that that you have to watch that for bias, but it’s still a ways in which they can use the data to provide it to the practicing clinicians to help them close care gaps. Etcetera. So you know there are administrative uses. There are ways in which to, you know, sort of prepare some information for clinical uses. It sort of runs the gamut.
Speaker 3
Yeah, I think that’s so right. It’s you asked me where we’re using it everywhere. Well, everywhere has the potential. So any business process we’ve got can be improved, enhanced by AI in some way, especially with the LLM’s, where now they are getting into tasks where we haven’t traditionally considered them as being active, but making any kind of prediction on the financial side around. Costs identifying members who would benefit from a particular solution or some care coordination case finding. Additional application of it. We also work in a valuative sense, so we need to know about how these solutions that we give to our Members are performing, because we want the best group, and we actually use AI in that as well. And on the provider side, because I’m like health for anyone that’s not familiar with us, we’re an integrated finance and delivery system. So we’re payer and provider working very closely with our provider arm on. I mentioned the ambient listening, a very exciting area where they can auto document. Patient notes also using that on both sides of the fence with kind of a customer services arm. So we can do not just live documentation. So the customer service Rep is able to talk to the member rather than having to think about what they’re writing down. And by the way, we get better quality data on what the Member actually called us about. But also doing live prompting and it can suggest things that we should talk about and. One of the. Big gains we’re seeing at the moment is speed to proficiency. So if you think about when you start a new job. you’ve got the human skills because you’ve been hired for it. You’re used to doing it, but what you don’t know is the organization and like the policies and the documentation, AI is OK at the human side, but really good at finding the relevant bits that you need to know. So you can combine the two things and you can serve up the right information very quickly. And that means a much more consistent. Much better quality of service and I think the thing that we’re really interested on the clinical side is how can it improve diagnosis and treatment. Those are very big, weighty issues, but are rightly tightly regulated by stuff like.
Speaker 2
You’re my dreams come true later on.
Speaker 3
We’ll do that, but I think.
Speaker 1
What? What? What is your dream?
Speaker 2
Yyou know, back to my example of having autoimmune disorders, right? And no one sort of knowing like, well, we know you’ve got something else, but who knows what it is, right. So you’re not going to do that without big data and an ability to look at sort of broader populations. You’re not going to figure it out with your the end of one in front of one physician.
Speaker 3
Yeah that’s huge potential I think The next five years, maybe three years. Everyone’s gonna go for the low hanging fruit, right? It’ll be the operational stuff, the admin stuff that we take out because it’s relatively safe to do that. We need to be cautious obviously, but I think it will be a longer time with a lot more research before AI becomes really heavily impactful within clinical decision making.
Speaker 1
Because that’s not where it is now. Would you agree with that? The issues of AI scribe ambient listening, scheduling, those aren’t about diagnosis and treatment, where the real value is about operational efficiency, so.
Speaker 2
I don’t. I don’t know that I don’t know that 100% agree with that though, because when you think about scheduling back to the point of quality is not just the care you got, but potentially the care you didn’t get right. So some of it is if we can create more. Efficiencies where we know they’re going to be fewer missed appointments or such or looking at those sorts of things. Again, some of the administrative stuff can be both as beneficial and as risky, right? If it backfires and you’re finding that there are certain patient populations who aren’t getting in because the way the scheduling algorithm is written, which has happened, but it could be hugely beneficial if you’re getting people into care who otherwise wouldn’t. Have.
Speaker 4
Those administrative tasks. So we AMA does lots of surveys of physicians and our Members and and 20% of practicing US physicians today are using AI in their practices, but it’s administrative tasks, it’s back end office operations, scheduling, managing. Pay interactions, those kinds of things. The clinical applications which get me excited right when I think about how do you scale the capacity of the workforce are coming. The biggest challenge that we’ve got now is is really actually not on the development side. They’re really exciting tools out there. There are lots of now approved products on the market that you can buy, they can diagnose diabetic retinopathy. Very. Effectively right, using machine learning and data that’s been developed, the challenge is they’re one off point solutions and no CMIO wants to integrate 300 different point solutions across specialties. Impossible, right too timely, too expensive. You can’t manage it. So until there is a platform approach where these point solutions. Going to be plugged in, we’re going to be sort of at this juncture where it’s really challenging to actually implement at scale. And I also worry about, you know the, you know, 50% of care that’s delivered in small practices across the country. Now, there’s been a lot of consolidation. That trend is not going away. Say, but small practices independent practices are still the backbone of the delivery system, right? And having a capital stack or the sophistication to implement these tools does not exist in a lot of those places. And what I don’t want to see happen is another digital divide across practice type where they don’t have access to these kinds of tools that can be important for delivering care to patients.
Speaker 1
How do you temper that enthusiasm that you have for the excitement of these tools to perhaps diagnose care more effectively or accelerate care balancing it to make sure that you mitigate risk? That you mitigate bias, some tools could detect something which we often refer to as a, you know incidentaloma in terms of we see something, but it doesn’t really have any significance. And then we subject the person to additional tests. How do you factor that into your adoption of AI tools?
Speaker 4
I mean, it’s like any new innovation in medicine and you know, the AMA got its start in the 1800s foundationally with our ethical policy, right. What is the ethical policy need to be for the practice of medicine stamping out snake oil and quackery and we have long standing policy around what should be required for innovation to be adopted. When should innovation become the standard of care, right? What should we require as professionals to make sure that we are doing the best thing possible for our patients? What I would say though is if if we’re not very intentional around having a framework to ensure that we don’t allow bias to creep into data sets. That causes patient harm for underrepresented or marginalized populations. It will happen, right? So you take the example of the pulse oximeter and you all know the story, right widely described during COVID. That pulse ox today, the one I used on Monday is miscalibrated still today for patients with dark skin tones. This is not new. We’ve known about it for 30 years. Nobody has ever felt it’s been important enough, urgent enough to act on that until finally. Now there’s work at the FDA to figure out how do we label that, which I’m appreciative. Of if we’re not very intentional with these AI tools, that problem will happen over and over and over again in insidious ways that we won’t detect. And so the AMA is very vocal about wanting to make sure that we’ve got the right framework to demand that we think about these issues up front. We have something that we’re part of called the in full health initiative, which is how we make sure that these innovations. Are designed for all communities with input from those communities to make sure that you know venture capital is available to people from those communities. To ensure that we have things that actually work for all of the needs. But if we’re not very explicit about doing those things, we’re going to see the same cycle happen over and over and over.
Speaker 1
Now on this stage we all have enthusiasm for AI, but we also have to acknowledge there are many people who are not as enthusiastic perhaps in our organizations. They may be fearful of it because they really don’t understand it, which can be challenging or often in healthcare. We might have a wait and see approach, right? We’ve heard about a lot of other things EHR, others that we’re going to make doctors lives easier and healthcare better. So I’d like each of you to talk about within your own organizations how have you addressed the hesitancy, perhaps of your colleagues in adopting some innovative technologies as it relates to AI, do they say, Danielle not not today like let let let’s wait and see. Let, let’s give it a little more time to mature or do you say yeah let’s go aheaad.
Speaker 2
You know it’s it’s. Interesting because you know AHIP as an as an association, right? We represent all the payers nationally. But internally we don’t really use AI as as an organization, right? And part of it is, do you want to start using it before you have a robust enough framework, right? The same conversation at the national level has to happen within each organization, right? And so I think part of the, you know, what we’ve seen with our Members. Around, you know, risk and other things where that’s one of the things that stops people, right? you wanted to clear the hurdles, you know, part of it is going through these sort of big governance, you know, sort of taking your data governance that you had before. But looking at it with this sort of fresh new territory. And setting those parameters and how is this going to work? And it’s not just about the tech people. It has to have legal people and clinical people and others involved in the discussion. And so I think sort of setting out and thinking through like, here’s your plan, what are we going to do if and when these certain things go wrong, starts to help give people more comfort. That you know that structure gives them comfort to move forward.
Speaker 1
Do you have a Chief AI officer?
Speaker 2
we do not.
Speaker 1
Who here has a chief AI officer at their company?
Speaker 2
I mean, some of our plans do I will say that, OK, several of our plans do.
Speaker 1
so not too many, and I should point out the Department of Health and Human Services and now calling for the creation of a Chief AI Officer. But Ian, at your organization, how do you address some of this? Trepidation or hesitancy about in healthcare. Often as it relates to this, you don’t necessarily want to be first. Do you?
Speaker 3
Yeah. Yeah, that’s definitely true. And it’s a really. interesting dichotomy within our our workforce, sometimes within the same individual of being really excited about potential of AI and just give me give me the tools I need and I can do all this stuff with it and versus obviously people fearing for what it means for them. And I think up until I know couple of years ago. Say in the organization doesn’t use AI. I think that was. Yeah, I I believe that you needed like a set use case. You need the data scientists like me and my group who understood how this stuff worked, how to put it together. And it was pretty unlikely someone would be doing that kind of without attracting the attention of the organization because of the systems they needed to use. Of course, with large language models and being available publicly on the Internet, now everyone’s got access. So yeah, even if there’s no official organizational. AI service it’s entirely possible that within all of our organisations, people are getting on their phone, going to ChatGPT perplexity and putting their stuff in because they want to use it. They see it as an advantage. So one of the things we did within Highmark immediately. They came out and blessed them. RIT. Guys spotted the potential Disclosiveness within open ChatGPT a couple of weeks before the Samsung incident broke. That was when a couple of again very well meaning software engineers at Samsung put their code into ChatGPT to see if they could improve it, not realizing with the right set of prompts. Anyone could then retrieve that preparatory code. So yeah, we’ve locked it all down. Made sure all of our staff were trained in AI what you could use these tools for what you can’t use those tools for absolutely no CSI PHI into them and then we set about giving people safe, secure access to these tools. So within high marks zero data loss environments, we use Google Cloud. So we’ve got a version of Google’s Gemini model which is totally safe. For people to use with their hi mark and everyone’s trained on how to use it. So that’s kind of what we do in terms of limiting the expectation, I think. Especially, you know the business leaders hear about AI, there’s a little bit of a kind of arms race of like everyone thinks everyone else is further ahead with AI. There’s some real practical challenges bringing it in. So I’d say, you know, in all of your organizations, think about. You know where it’s going to add the most, but also you know, be realistic about some of the time it will take to get one of these applications in production. But in terms of tempering people’s concerns about it, we just pushed this notion of human at the helm. So AI augmenting people’s workflow, taking away the easy parts, but letting them concentrate on the skills that we pay them for whatever their role is working at the top. Of their license. And the analogy.
Speaker 1
How? How is that response taking away the easy part?
Speaker 3
Well, I mean. There are probably some people that have a very. Easy routine and OK, that gives him pleasure. That’s an interesting debate, but the example we use is we’re still trying to figure out how big this wave of enthusiasm is it going to be a bubble that bursts or is it going to carry on? But it could be potentially as big as when the introduced desktop computers to the workplace in the 80s, that big and what we saw then. Was there were some roles that disappeared, like typing pools gone within about a decade, I think. But most roles carried on, they just did their job much more efficiently. Actuaries moved from pen and paper onto spreadsheets, and suddenly they were much more productive because they were using their actuarial skills in a better way and they weren’t spending time doing calculations by hand. And then a whole bunch of new roles appeared suddenly. Companies had to spin up IT departments. Look after all of these computers they just purchased, so you got to think what might those new roles be as we AI continues to change the way we operate within the workplace.
Speaker 4
You know, I’ve lost track of the number of EHR conversions and data mapping exercise that I’ve either driven, led or been a part of. And because of those experiences that have been bumpy in most of the circumstances, I think a lot of practitioners today still have a little PTSD. You know, we’ve gotten the HR. It’s gonna make everything great. You’re off paper and, oh, wait, it doesn’t have great usability. You have to change your workflow to use the tool that we’ve just given you that’s now been mandated by the organization. Not a great experience for a lot of folks and I I’m I’m an informaticist right? I’m trained in informatics. I believe in these technologies and yet I understand why, you know, through your tax dollars. Thank you very much. You know, we’ve incentivized probably a little too early. The adoption of health IT in a way that the rollout has caused some trepidation, I think amongst practitioners. And that’s why when we survey physicians, you know 40, 41% are equally excited as they are terrified about AI. And I think it’s because of that experience of the EHR rollout that you know was not always frictionless. What is this new thing going to mean? While there’s, I think, just general anxiety in society about, you know, how does it change roles? What does it mean for my relationship with my patients? How does that interaction differ? And I, I will tell you, you know, I I think there are lots of things when it comes to. Getting rid of the easy stuff. That has profound implications for roles and jobs and balance in life. And you know, I wasn’t planning to go here today. But you know, I I’m an anesthesiologist and and we are very firm believers in physician led healthcare teams. So we could have that discussion different day about scope of practice. But if you take. All of the really simple things away from me and I spend my day biting my nails, just doing the really, really hard stuff because maybe somebody else, a tool, a technology, a person can handle some of those other cases other patient needs. I’m left burnt out because I can’t get through my day biting my nails. Simply just doing the really, really, really hard stuff so that cognitive load balancing as we bring these tools into practice is things that some folks are only starting to really understand.
Speaker 2
I think there’s also much higher level risk that some of this builds up to in terms of. Of you know, a discussion as America’s right and Americans on the cost of the technology, right. Because there’s a balance between potentially paying more per service, right. And so you can provide fewer services and not burn out, right, or you know. Paying for these technologies that we know that there’s a big benefit for, but we also don’t want premiums to be so expensive that people end up not being able to afford it, right. So there has to be sort of a bigger conversation about how do we balance this and grow this in a way that that works for the overarching system.
Speaker 1
What if I could show you an AI tool? Let’s take imaging radiology. Where there’s a. Lot of data. It’s looking at patterns and and show you that if a radiologist uses an AI tool to help diagnose breast cancer and it can show. A A greater level of accuracy. Would it be improper for healthcare systems not to be utilizing this tool?
Speaker 4
There are already lawsuits in that space, I’m I’m aware of at least one where a patients family is suing a well known, well branded health system because they didn’t use an AI tool related to a clinical care process now. The the unfortunate thing for that patients family is the tool actually doesn’t exist, that they in the way that they think it does. So I think that the health system will be fine, but they’ll be this expectation, yeah, right. And and and and look the the standard of care is not defined by the capabilities, the standard of care is defined locally, right. And that’s why we have local oversight of through medical boards and nursing boards of clinical practice. That’s why we. have hospital and practice facility policies and so that standard will change and there will be a moment where innovation and technology becomes a standard of care. I think we’re far away from that, but certainly I I could imagine that, you know, every chest film in the country is primarily read by a machine and then over read by a radiologist. That that would be a reality that I think is probably. Not far off.
Speaker 2
You know all of this? I mean, there’s there’s so many things to think about from all this. It almost, you know, you know, makes you a little bit frightened, right. But the the from a payment perspective to part of what the payers are trying to figure out right in combination with how AMA creates codes and creates relative values and such is. You know, should things be bundled into a payment because it is assumed that it’s the standard of care? That it’s part of the underlying code or when is it separate? Because, but if it’s separate, does that mean only sort of high capitalized practices are going to end up using it or not, you know, so there’s a lot of dynamics that we don’t 100% sort of understand the behavioral economics and and you know. It’s not. The reality is, is that what maybe should be standard or not? It’s super Gray right now. There’s not. These technologies are not necessarily always like. You know whiz bang like 100%. Absolutely everybody should do this tomorrow. There’s more of that in between factor, so it’s going to be there going to be a lot of growing pains as we try to figure this out.
and these aren’t AI problems, these are standard of care problems. Our practice problems, access to technology problems.
Speaker 2
It’s integration of the technology into all of these other standard processes that we have, whether it’s coverage or payment or hair guidelines.
Speaker 3
We’ve lived with this stuff for years. I’m not saying weve found the best set of solutions. There might be better solutions out there, but it’s it’s not an AI driven problem. It’s how AI fits into the existing problem we’ve already. Got.
Speaker 4
And in our research, across any technology innovation, digital health tool, whether it’s AI enabled or not, there are four drivers of adoption. It’s does the thing work and and that becomes really hard when you’re talking about a new algorithm understanding, you know, when it maybe it’s not regulated and there’s no table one and FDA packet insert to understand what the tool is doing. The 2nd is payment, right? That’s really important. If you go through the acquisition cost of a tool, how does that get bundled into the service and paid for the third is liability. We haven’t touched much about this, but when something goes wrong, who’s liable, right? If I rely on an algorithm to decide a course of cancer treatment for a patient with breast cancer and it’s the wrong treatment. And I’ve relied on the algorithm. Who’s holding the bag for that? Right. Is it the developer? Is that the implementer? Is the person you know with the biased data set that should have recognized that there was a problem? Is it me, the end user, the clinician? I think there a lot of unanswered questions that we’ll have to sort of muddle through as these issues are sorted out and and the final thing is does actually work in my practice. And and I I will tell you, I’ve made this mistake. I will just admit it. Back in the day, I developed some software and I should know better because like I went to medical school. You know, and I have two children now. Maybe that was a problem I didn’t have children back then. You know, children are just not small adults. And and and and we built some software in our adult Hospital in Boston and we turned on the Children’s Hospital and it did not work. And we did not recognize that until some things happened downstream, the workflows were different. It had nothing to do with the Physiology about kids and adults. It was the workflows, the nurses. How things were documented and collected. I just didn’t recognize early in my career and understanding how you translate technologies that were developed in one setting into another and whether they do or do not work is the 4th driver of adoption.
Speaker 2
Sure. We don’t need you anymore. I’m kidding, developer yeah.
Speaker 2
I’m kidding. I’m kidding. Developer and deployers, right, I think, is an important piece also for the the payers as well. But you know just sort of tagging on from a a clinical example, right, the office of the National Coordinator for Health IT although that they just officially had a name change. So I think I’m supposed to say it’s assistant secretary for technology or something like that. Anyway, so you know they have this new policy around transparency of the use of AI within electronic health records. It’s, you know, some people kind of call it a nutrition label. Just so you understand what it is. Some people say don’t call it that, but just, you know, a conceptually, I think some of it is well, is that information enough? For a physician to really A have confidence and use it and B the you know the fear of the liability piece of is it understandable is it, is it clear or is it actually actionable and and Even so, I’m worried that when we get to this sort of like snapshot kind of perspective, we don’t know what we don’t know from the developers when we’re the deployers, right, so. We may be able to see what data sources they’re using, but we don’t know the five other data sources that maybe they could have used that they didn’t use and maybe would had a better product or something that’s less biased, etc. Because we, you know, maybe it was more expensive or something, right. So when we try to get down to these little snapshots, it’s it might be misleading to sort of look like ohh the the deployers maybe had some responsibility but but that some of this is really more upstream and aren’t necessarily things that. The DEPLOYERS can control.
Speaker 1
We have about 14 minutes to solve all of these problems and and I do want to point out you all started with all of your excitement about AI. So the flip side could also be in your example or the clinicians. That are not using AI that would have given a quicker diagnosis or more accurate diagnosis, a better therapeutic option. That’s where the real concern could be. But we’re at the Alliance for Health Policy. We’re in Washington. It’s all about policy, right. So what’s the one or two policy initiatives? Danielle and Jesse that you two are working. On your organizations as it relates to AI and health and then I’d love to hear from Ian, should the government be involved in this or should we just really be allowing industry to lead?
Speaker 4
Yes. They should be.
Speaker 1
But let’s let’s let’s hear the the.
Speaker 4
Yeah. Look, we we need a.
Speaker 1
Initiatives the policy initiatives. That the AMA is working on.
Speaker 4
We need, we need a national governance framework. And, you know, given the state of current old future regulations maybe being a little bit up in the air these days, that may require congressional action. I I don’t know. But we we need standards, we need regulation. It shouldn’t happen a way that stifles innovation and puts the brakes on all of these things. But we’ve got to have clear standards if we’re going to manage some of these issues that we’ve been talking about. Related to liability acceptability, training, usability as well as does the thing actually work, you know? And as a clinician, I should be able to know if the thing actually works, but that’s not going to happen in a uniform way if we don’t have some standards.
Speaker 1
the government’s going to tell you that it works, that’s who you’re relying upon.
Speaker 4
Well, look, what’s the FDA’s role to make sure we have safe and effective products in the marketplace and not all these products will be mandated, but the FDA’s framework as it is today does not work to look at individual algorithms and individual software changes at scale. They could never hire enough people to do that, so there’s got to be something different, right? And does that require you know, some congressional change in the law? Does that require FDA? I I don’t know. We have thoughts about it. The AMA has been working on AI policy for six years. It’s not new to us we did do a major refresh last fall. Our AI policy is freely available for downloading a Nice PDF on our website, and I would encourage folks to check it out. If you’re interested in our transparency and other principles as it relates to this, it’s a a pretty easy document with an executive summary.
Speaker 1
All right, Danielle, what’s what are you working on? That’s gonna solve these problems? What policy initiatives?
Speaker 2
So you know, I mean I think we we have sort of several fronts, right, we’ve, you know, to the point of we’ve been working on this for years. Working on this in the States for far longer than we have at the federal level. Done a lot of work with the NAIC, the insurance commissioners around the country on building principles and guidelines and individual states have various bills. It was super interesting that Colorado recently put in a new bill and the governor. Put out a statement with it basically saying. I don’t think we should be doing this. I’m paraphrasing a little extremely, but it’s, you know, like this is something that I think really should be more of a federal. There should be some federal parameters and then some state, you know, on top of that. So it’s still interesting, it’s going to be interesting to see the interplay and the notion of like sort of state preemption like what is at the federal level versus what’s at the state level. You know, certainly the executive order on AI was enormous and the the sort of DC urban legend is, is the longest executive order ever. I don’t know if anybody’s going to check the page count or not, but. But that’s really like a whole of government set of changes and you know, a lot of it is around like how do we stay ahead of China and you know some of that kind of stuff. But there’s a lot of healthcare in there and we we don’t we haven’t seen yet how that’s going to cascade. There’s been doing that. You know HHS has been doing a lot of internal things like announcing their new structure. New personnel and such, but we kind of went backwards, right, like we we were working at the like NIST level like Super Uber detailed standards level before we did kind of like the principles and the high level frameworks. And so we’re kind of awkwardly going back to the top and then trying to go down to the bottom. So we’re kind of missing that level of like, what does this actually mean in the various regulations? That we have to comply with and they’re kind of filling those back in. So I think there’s going to be a lot more of that over over the next several years. In the meantime, we have to comment to every alphabet soup of OST PFTC nest CMS OC. Etcetera. But on the congressional side, obviously there’s a lot more interest this past year. There’s a lot going on at sort of the high levels. I think you’ve all seen, you know, Senator Schumer, like meeting with all of the big tech companies and sort of splashes in that way. But the question is like how is healthcare going to be different, right. You know, I. Sometimes I say in, you know, telling using AI to tell you if you have cancer or not is more risky than making you turn left instead of right. But now that I know your hedge row example, maybe the left and right is also very dangerous, but I think you get you get my drift right? Like some of some of the simple algorithms are different. Then some major analytics. So you know at the congressional level, we have some of these issues around the liability around balancing innovation and consumer protections, risk based approaches where you’re putting more emphasis and review on the higher risk items. Using the existing law base we don’t need, we can’t discriminate, we don’t discriminate. We don’t need a new law for that. We need to be explaining how AI fits into those laws, right? So there’s not duplication and confusion and and.
Speaker 1
We need to update HIPAA?
Speaker 2
You know, I think privacy privacy sometimes gets conflated. I think the two are important together, but they aren’t equal, right?
Speaker 1
What’s a covered entity?
Speaker 2
So I think from our perspective privacy, you need to have more robust privacy law at the same time as AI, but some of the AI is not PHI, not etcetera. And it’s not a privacy risk. So we just have to remember it’s it’s an overlapping venn diagram. And we need the privacy changes and from our perspective, we think there should be more of a level playing field. I mean this has sort of come up in the notion of the interoperability rules at CMS where payers have to release information to third party apps on behalf of patients once the patient says yes, please send that data. It is no longer HIPPA protected. Your entire clinical record that the that the payer has. Can now be sold at an individually identifiable level, completely legally, right? So we think there is a big massive hole that you could drive a truck through in terms of our privacy framework.
Speaker 4
And there was an opportunity, unfortunately, that the federal government did not take to make that more transparent to consumers. When they are opting in to share that data and there’s there’s one policy opportunity right there that could certainly solve it. Executive order helpful, not sufficient, right. It’s a road map for some of the things we need to do around patient privacy protection. And obviously there’s a lot of anxiety these days about things. Like cyber security attacks, given what we’ve been on, been living through all these data breaches and other challenges, and I think we’re going to solve some of those things again, through regulation.
Speaker 1
and is there an industry that you could point to that you think is utilizing AI well where the tool so some people will say? That the travel industry is using AI well, particularly generative AI, you can go into ChatGPT, say, find me a hotel, you know in Paris that’s close to the Eiffel Tower. That’s still reasonably priced during the Olympics. And give me a five day. You know itinerate and it can do all that in a matter of seconds. I I don’t even have to search anymore on Expedia orbits or whatever. So is there any industry that you’d point to say that healthcare needs to move more in that direction?
Speaker 3
I don’t know. I think healthcare. Industry is pretty special, right? So the examples you raised, yeah, they’re good. Any one, any company that exists as a website? Obviously, this is wonderful stuff for them. Yeah, but they’re pretty narrow. Like the travel agency. Yeah. All I’m doing is I’m looking for a flight, a hotel. It’s a recommendation. Engine. If I recommend a bad hotel or a bad flight, it’s not the end of the world. No one would call me. Well, you hope. But yeah, I think healthcare obviously very different. Literally life or death decisions that we’re influencing incredibly complex system, the human body, the amount of stuff, the amount of data we can capture on it, very limited. So what we’re using a bit of decisions, it’s a very different world. I I do think you know, Healthcare is traditionally risk averse quite rightly, but I also it’s made some I think really good progress on bringing in AI techniques with the appropriate level of caution over the last say decade or so.
Speaker 4
I mean, come back to Danielle’s problem. And by the way, to solve your dream, become an anesthesiologist, right? You know, we’re seeing really amazing things in the pharmaceutical development space using AI tools to rapidly accelerate drug discovery for novel targets that would never have been possible at the scale at a reduced cost at a time frame. Using some of these tools. And I think that’s going to open all sorts of really interesting avenues into developing new therapies and new tools, we just have to make sure we have an underlying healthcare system that can actually deliver those to patients.
Speaker 1
You all represent different types of organizations. So in our in our final, you know remarks, what’s your advice to executives and organizations like yours in terms of how they can think about utilizing AI, how they should think? About utilizing AI. In their operations.
Speaker 2
Who wants to start?
Speaker 4
I mean for for us internally it’s it’s not like are we going to use it? It’s how are we going to use it? How are we going to manage it and then you know how many use cases are there and how they integrate into business processes for our members, right? We have a ton of information, educational resources, toolkits, things that we do as an association. To make sure that physicians are getting the best information that they can, so is they’re trying to understand integration, to practice and questions that they’re getting from patients like my mother. You know, what is this thing? Should I be using it? They’re prepared to answer them.
Speaker 1
OK.
Speaker 3
I I’d definitely say, I mean I do this everyday. I advise our leadership on it, but it focus on the use case, right? It’s not the technology. You don’t need AI, you need to understand the problem you’re trying to solve. AI quite often can be a solution for that, but it is focused on how it’s going to be used and that sets you up in the right way. First of all, you’re thinking about the responsible AI aspects. Do I want to use AI in this context? What are the potential pitfalls? Then you go to do I have the data I need to solve that problem, which again is another big blocker. For these, we don’t have access to the data, it’s not joined up. We’re not capturing the right things. And then it’s about being able to monitor the success and adoption of the solution. We see lots of wonderful proof of concepts that just don’t work in practice. For whatever reason, so it’s not just having your model built, putting it in and then hoping people use it, it’s. Out helping them understand what it does, what it can do, what it can’t do, encourage them to use it if they need it, and always monitoring what’s going on for ways you can possibly improve what you’re doing.
Speaker 2
Yeah, I mean, I I think Ian said it well. You know from a health plan perspective, you know, I think a step 0. Right. Is the is the governance piece and making sure that you sit down and are intentional about how you decide what goes forward and you know, I think it’s, I think I said this earlier, but I think it’s really important to emphasize that it, it has to be a team that’s across disciplines because everybody needs to bring their different lens to it to really be able to identify where some of the problems may be and be able to, you know, course correct if those if those problems occur. But the monitoring is the key piece, I mean. But you know from my perspective as an association, right, I think all of our Members need to be really watching what’s coming from the congressional level, the state level plus the the new regs and the standards. And so it’s a lot. To keep up with, they’re going to have to come up with processes to make sure that these new compliance areas, they’ve got the robust business processes to keep up with sort of all this extra that’s coming.
Speaker 1
Jesse, Ian, and Danielle want to thank you for sharing your insights today. Thank all of you for listenting.